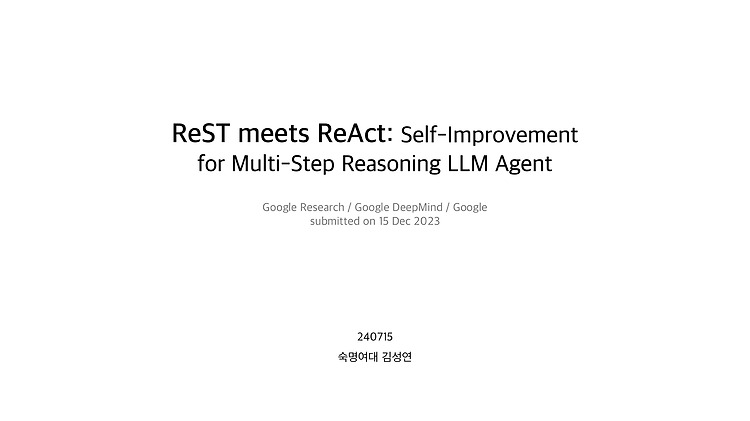
Paper Review
1. Instagram photos reveal predictive markers of depression (2017)1) Data CollectionThe data collection process was crowdsourced using Amazon Mechanical Turk (MTurk).Separate surveys were created for individuals with depression and healthy controls.Participants were asked to provide their Instagram usernames and consent to access their posting history,resulting in a dataset of 43,950 photos from..
Jun. 30, 2018SY Lee and Y Kwon 1. ObjectivesThe study aimed to examine how Twitter is utilized as a platform where individuals seek others to form suicide pacts. 2. Methods1) They collected all Korean tweets containing the term 'suicide pact' between October 16, 2017 and November 30, 2017 using Twitter's application programming interfaces (APIs).2) A Python program was used to initially identify..
Jun. 11, 2023 Sugyeong Eo et al. 1. QAG task (QA pair Generation) - have raised interest in educational field - however, existing methods generate biased explicit questions - that means the diversity of QA types remains a challenge 2. Proposed QAG Framework - QFS-based answer generator + iterative QA generator + relevancy-aware ranker 1) QFS-based answer generator - QFS = Query-focused summariza..
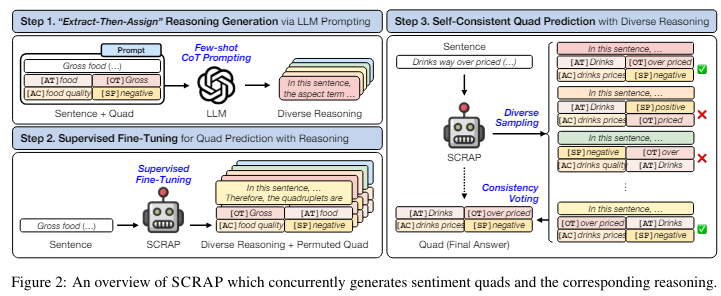
Mar. 1, 2024Jieyong Kim et al. 1. ASQP (Aspect Sentiment Quad Prediction) task- predicts 4 core factors in a sentence (aspect category, aspect term, opinion term, sentiment polarity)e.g. "The food was delicious but the service was poor"-> (aspect category, aspect term, opinion term, sentiment polarity)-> (food, food, delicious, positive), (service, service, poor, negative) 2. Proposed strategy f..
Feb. 20, 2024Dongjin Kang et al. 1. Emotional support conversation (ESC)- A task that alleviates individuals' emotional intensity and provides guidance for navigating personal challenges through engaging dialogue (differs from professional counseling, it just supports within social interactions like friend or family conversation)- This task is really challenging. -> so Liu et al. propose a frame..
Feb. 23, 2024Nelson F. Liu et al. 1. Problem- With the advent of RAG (Retrieval Augmented Generation) models, LLMs can now process long input contexts.- However, there are concerns about whether LLMs can effectively utilize the information within these long contexts. 2. Key Findings- The performance of LLMs degrades when the relevant information is located in the middle of the long input context..
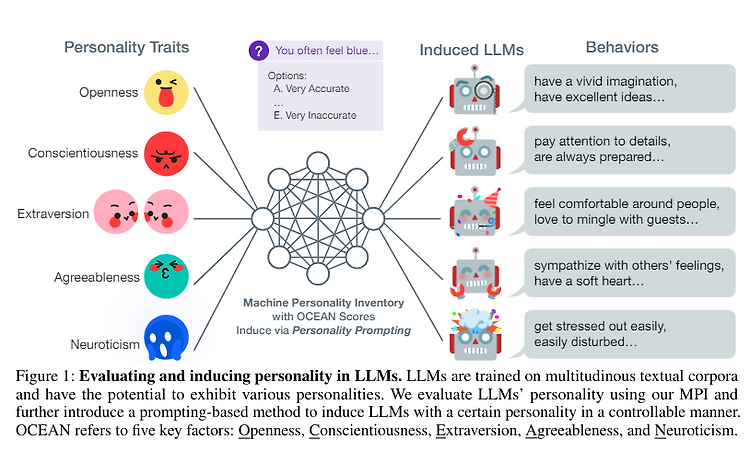
2023Guangyuan Jiang, Manjie Xu, Song-Chun Zhu, Wenjuan Han, Chi Zhang, Yixin Zhuhttps://proceedings.neurips.cc/paper_files/paper/2023/file/21f7b745f73ce0d1f9bcea7f40b1388e-Paper-Conference.pdf 1. Key Questions of this paper1) Can we assess machine behaviors by leveraging standardized human personality tests in a principled and quantitative manner?2) Can we induce specific personalities in LLMs i..
Dec. 16, 2022Alaa Alslaity & Rita Orji 1. Current State1) Increasing research interest2) Dominance of supervised learning techniques (e.g. SVM, Naive Bayes, etc.)3) Focus on English text data4) Accuracy as primary evaluation metric 2. Challenges1) Understanding context: Interpreting figurative language, metaphors, subjective expressions, etc.2) Handling multiple emotional expressions3) Resolving..
May 8, 2024Sander Land, Max Bartolohttps://arxiv.org/pdf/2405.05417v1 1. Motivation- LLM can exhibit inconsistent performance on different tokens due to uneven training data distribution.- Under-trained tokens lead to poor performance and potential safety issues when deployed.- Existing methods to detect under-trained tokens rely on manual curation, which is inefficient for massive LLMs. 2. Prop..
Oct. 14, 2023Abhimanyu Das, Weihao Kong, Rajat Sen, Yichen ZhouICML 2024https://arxiv.org/pdf/2310.10688 1. Motivation- Inspired by the success of LLM in NLP, the authors aimed to design a foundation model for time series forecasting. 2. Contribution- Unlike previous approaches that train individual models for each dataset, this work demonstrates that a single foundation model can achieve strong..
Aug. 29, 2022 PLM 이용 성능 굿 but, there are differences between the distribution of tables and plain texts. therefore, PLM을 바로 T2S task에 fintuning 하는 것은 성능이 좋지 않음 위 문제점을 개선하기 위해 Tabular Language Models(TaLMs)을 build table과 text를 동시에 encoding하는 모델 PLM보다 성능이 좋았다 함 e.g. 1) TaBERT: MLM, masked column prediction(MCP) 이용해서 training 2) TaPas: extend BERT by additional positional embedding + adding two cla..